AI-Ops Framework for Automated, Intelligent and Reliable Data/AI Pipelines Lifecycle with Humans-in-the-Loop and Coupling of Hybrid Science-Guided and AI Models (AI-DAPT)
Visually-hidden
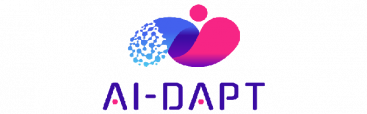
Today, Artificial Intelligence (AI) has paved a long way since its inception and has started experiencing exponential growth across various industries and shaping our world in ways that were once thought impossible. As AI transitions from research to deployment, leveraging the appropriate data to develop and evaluate AI models has evolved into one of its greatest challenges. Data are in fact the raw material and the most indispensable asset fuelling much of today’s progress in AI, generating previously unattainable insights, assisting more evidence-based decision-making, and bringing tangible business/economic benefits and innovation to all involved stakeholders. However, despite their instrumental role in determining performance, fairness, and robustness of AI systems, data are paradoxically characterised as the most under-valued and de-glamorised aspect of AI while a data-centric focus is typically lacking in the current AI research.
AI-DAPT aims to deliver an innovative and impactful research agenda that will provide tangible benefits to a variety of stakeholders that struggle with making AI services. Seeking to reinstate the pure data-related work in its rightful place, and reinforcing the generalizability, reliability, trustworthiness, and fairness of Al solutions, AI-DAPT vision relies on the implementation of an AIOps framework to support and automate AI pipelines that continuously learn and adapt based on their context. It enables proper purposing, collection, documentation, (bias) valuation, annotation, curation and synthetic generation of data, while keeping humans-in-the-loop across five axis: (i) Data Design for AI, (ii) Data Nurturing for AI, (iii) Data Generation for AI, (iv) Model Delivery for AI, (v) Data-Model Optimization for AI.
Project Website: https://www.ai-dapt.eu/project/